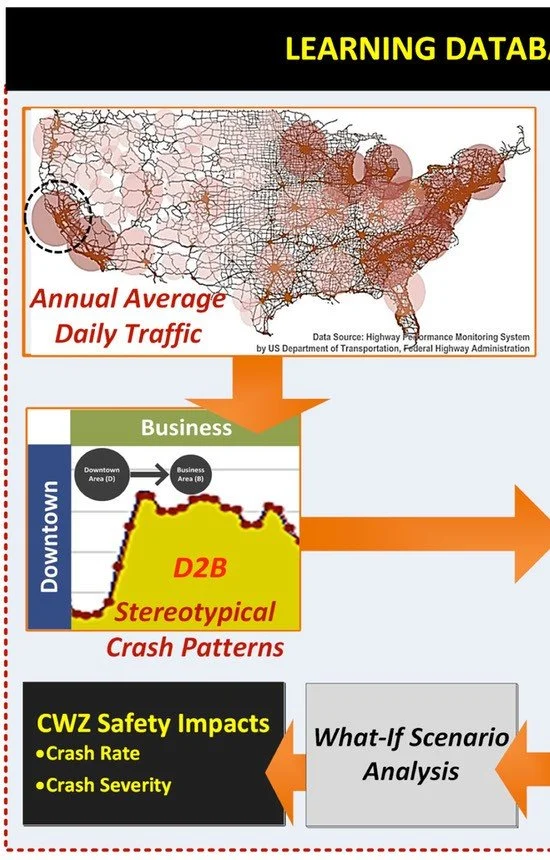
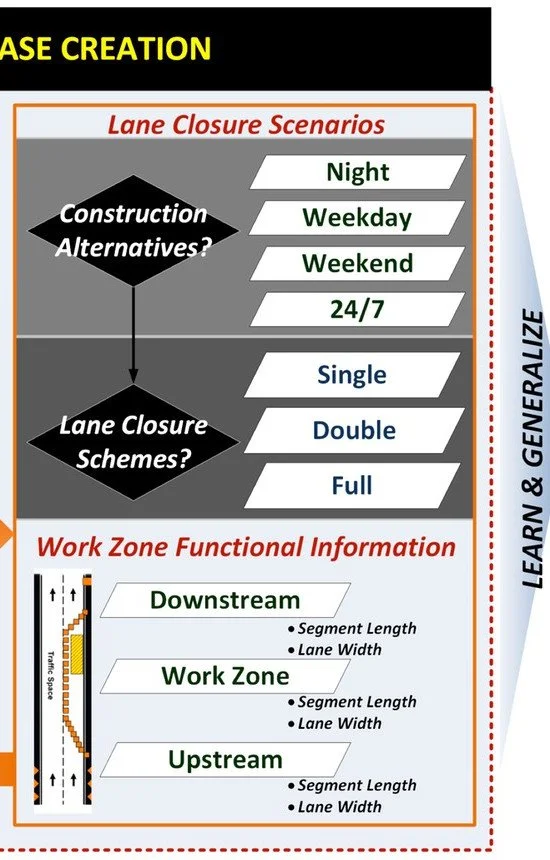
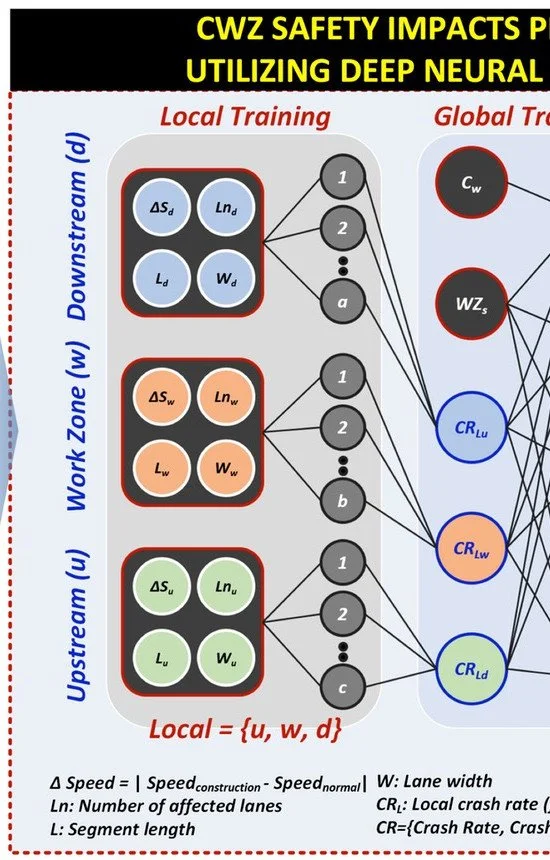
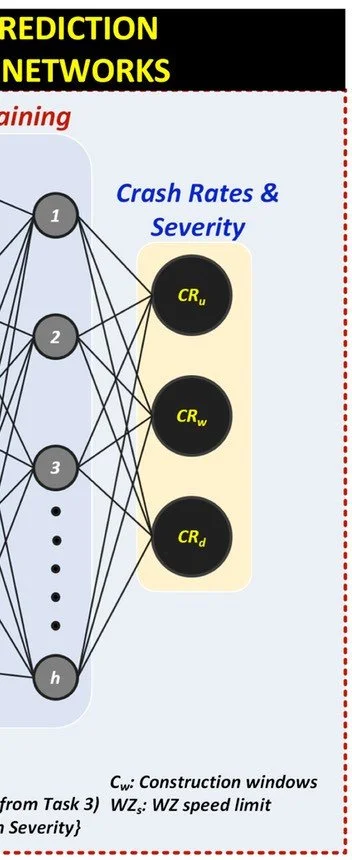
SRP2: Autonomous Crash Risk Prediction of Highway Construction Work Zones
Using sensor-based big data in transportation planning has become increasingly common, yet a critical knowledge gap concerning the data’s precision persists. Even in a common spatial region, significant variations exist in data collected before and during construction due to the lack of spatial correlation between neighboring sensors within a road section. Furthermore, a new federal rule requires all state transportation agencies to implement work zone safety impact assessments. These impact assessments are critical but also challenging and expensive to produce, with the primary problem being a lack of standardized methods. No methods or tools mirror work zones' unique spatiotemporal dynamic characteristics, and the typical techniques are often project-specific, frequency-driven, time-consuming, costly, and error-prone. This project fills these two knowledge gaps by providing a novel big data imputation method and, ultimately, creating a data-driven decision-support model and testing its reliability for predicting accident risks. Therefore, this project's primary objective is to create a big-data-based computer model and test its reliability for predicting accident risks caused by the presence of a work zone under arbitrary lane closure strategies. This will be achieved by conducting a five-stage method that articulates a new spatiotemporal data imputation technique and modeling frameworks where the level of safety disruption is assessed and the model’s prediction accuracy, fused from a machine-learning algorithm, is validated.